Copula-based multivariate association measures and tail coefficients
Mnohorozměrné míry asociace a koeficienty závislosti chvostů založené na kopulích
dissertation thesis (DEFENDED)
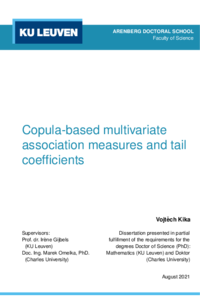
View/ Open
Permanent link
http://hdl.handle.net/20.500.11956/173883Identifiers
Study Information System: 190243
Collections
- Kvalifikační práce [11216]
Author
Advisor
Referee
Veraverbeke, Noel
Fuchs, Sebastian
Faculty / Institute
Faculty of Mathematics and Physics
Discipline
Probability and statistics, econometrics and financial mathematics
Department
Department of Probability and Mathematical Statistics
Date of defense
10. 9. 2021
Publisher
Univerzita Karlova, Matematicko-fyzikální fakultaLanguage
English
Grade
Pass
Keywords (Czech)
asymptotická normalita|konzistence|kopule|míra asociace|mnohorozměrná analýza|odhad|shlukování|závislost|závislost chvostůKeywords (English)
association measure|asymptotic normality|cluster analysis|consistency|copula|dependence|estimation|multivariate analysis|tail dependenceStruktura závislosti d-rozměrného náhodného vektoru X je obecně složitý koncept, který je plně popsán sdruženým rozdělením tohoto náhodného vektoru. Co se týká samotné závislosti, tak se lze zaměřit pouze na příslušnou kopuli vektoru X, která nebere v úvahu marginální rozdělení jednotlivých složek X, ale stále plně popisuje jeho strukturu závislosti. Kopule je definována jako funkce na d- rozměrném intervalu [0, 1]d s hodnotami v intervalu [0, 1]. Kvůli tomu může být příliš složitá pro praktické použití, neboť uživatelé v praxi zpravidla preferují jednodušší ukazatele, které vhodným způsobem shrnují informaci o struktuře závislosti zachycené kopulí. Takovým jednodušším ukazatelem může být vhodná míra asociace (korelace), neboli hodnota, která popisuje tendenci složek vektoru X nabývat zároveň velkých, nebo malých, hodnot. Koeficienty jako Kendallovo tau nebo Spearmanovo rhó, které měří sílu asociace mezi dvěma náhodnými veličinami, byly důkladně studovány a popsány v polovině 20. století. Požadavky na dvourozměrné míry asociace jsou tak již dobře známé. Zobecnění takových měr do vyšších dimenzí ovšem není přímočaré a přináší otázky ohledně jejich žádoucích vlastností. Často lze také míry asociace do vyšších dimenzí zobecňovat různými způsoby a není na první pohled jasné, který z těchto způsobů preferovat....
The dependence structure of a d-variate random vector X is a very complex notion which is fully described by the distribution of the random vector. Alternatively, it suffices to look into the corresponding copula function of X, as it ignores the marginal distributions of X but still fully describes the dependence structure. However, a copula is a function defined on the d-dimensional hypercube [0, 1]d with values in the interval [0, 1]. As such, it might be too complex for practical use and one would prefer to have tools that can translate the information from the copula function into a simpler indicator. In particular, of interest might be an association measure, that is, a single number that describes the tendency of the components of X to simultaneously take large or small values. Coefficients like Kendall's tau or Spearman's rho, used to measure (strength of) an association between two random variables, were thoroughly studied and described in the middle of 20th century. Requirements on bivariate association measures are well-known. However, generalization of such measures into higher dimensions is not very straightforward and brings discussion on the desirable properties. In addition, bivariate association measures can be often generalized in multiple manners. The same holds true if one wants to...